How Fault Learning and Digital Twins are Revolutionizing Data Validation
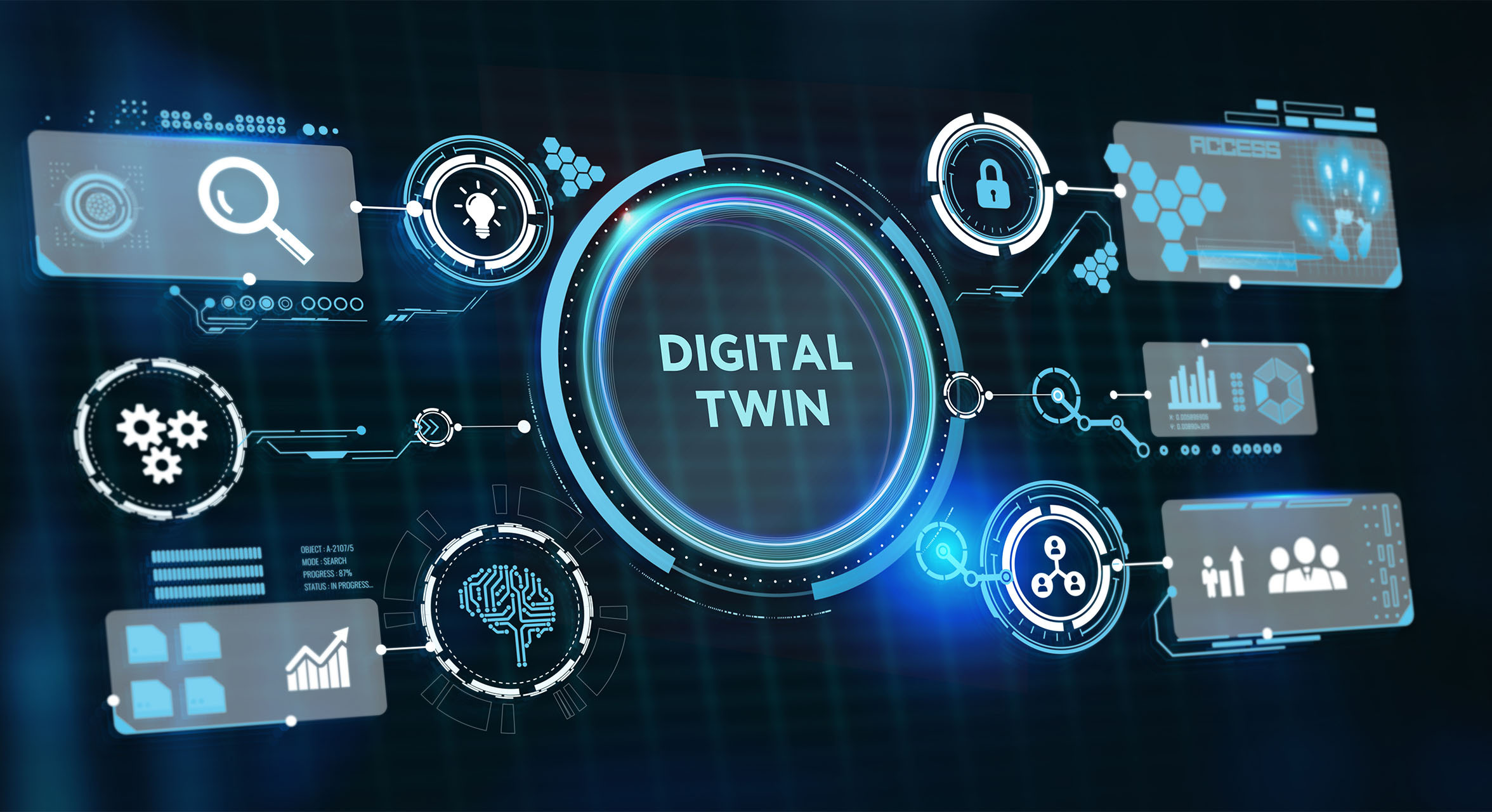
Data validation is a critical yet often overlooked aspect of manufacturing data collection. Accurate validation ensures that production data is reliable, actionable, and capable of driving meaningful process improvements. Traditionally, data validation has been a manual and labor-intensive process, requiring engineers to compare logged data with real-time events, identify inconsistencies, and make iterative adjustments. This approach is time-consuming, costly, and prone to human error. However, advancements in machine learning and simulation technologies—particularly Fault Learning and Digital Twins—are transforming the way manufacturers validate and utilize data.
The Inefficiencies of Traditional Data Validation
Historically, manufacturers have relied on manual observation and intervention to validate production data. Engineers must assess downtime logs, cross-reference system data, and adjust inputs to ensure accuracy. While this method has been the industry standard, it presents several inefficiencies:
- Time-Intensive Processes
Manual data validation is a slow process. Engineers must wait for production lines to stop before they can compare downtime logs against Manufacturing Execution Systems (MES) or Overall Equipment Effectiveness (OEE) data. This delay in validation prolongs the time it takes to identify and address issues, leading to extended downtime and lost productivity.
- High Costs, Especially in Regulated Industries
Industries with strict compliance requirements—such as pharmaceuticals, food and beverage, and medical device manufacturing—incur substantial costs in ensuring data integrity. Revalidating systems after software updates or operational changes can cost hundreds of thousands to millions of dollars. These expenses can escalate further if inaccurate data leads to non-compliance penalties or product recalls.
- Data Inconsistencies and Subjectivity
When data validation is done manually, discrepancies are common. Operator input can be inconsistent due to subjectivity in identifying faults and logging downtime events. For example, one shift operator might classify a machine stoppage as a “mechanical failure,” while another logs it as “operator error.” These inconsistencies make it difficult to draw accurate conclusions about production efficiency and reliability.
Introducing Fault Learning for Smarter Validation
To overcome the limitations of manual validation, manufacturers are adopting Fault Learning, an advanced approach that leverages machine learning to automatically detect and categorize faults based on real-time machine activity. This method improves efficiency and ensures consistent, high-quality data validation. Key benefits of fault learning include:
- Reduced Engineering Effort
Machine learning algorithms eliminate the need for engineers to manually tag and categorize faults. Instead of relying on human observation, the system autonomously detects patterns in machine behavior, significantly reducing labor-intensive validation efforts. This automation allows engineers to focus on higher-value tasks such as process optimization and predictive maintenance.
- Dynamic Fault Prioritization
Not all faults have the same impact on production. Fault Learning systems rank issues based on frequency and cumulative downtime, allowing manufacturers to prioritize corrective actions effectively. By addressing the most critical issues first, manufacturers can significantly improve uptime and operational efficiency.
- Continuous Learning and Adaptation
Traditional validation methods rely on static rules and predefined fault categories, which can quickly become outdated as production environments evolve. Fault Learning systems continuously update their fault detection algorithms, ensuring that new and emerging issues are automatically incorporated. This adaptive approach enhances long-term data accuracy and reliability.
Digital Twins: A Game-Changer for Data Validation
While Fault Learning improves real-time fault detection, Digital Twins take data validation a step further by providing a virtual environment for analysis and simulation. A Digital Twin is a highly detailed, dynamic replica of a physical production environment, allowing manufacturers to analyze past production events, simulate future scenarios, and validate process changes without disrupting operations. The advantages of using digital twins for data validation include:
- Simulated Production Sequences
Digital Twins allow engineers to replay and simulate production events to test different scenarios and potential solutions. If a machine failure occurs, the system can rewind data and analyze what happened before, during, and after the event. This capability eliminates the need for physical trial-and-error experiments on production lines, reducing downtime and associated costs.
- Enhanced Root Cause Analysis
Traditional validation methods rely on manual observations and limited historical data, making root cause analysis difficult and often imprecise. Digital Twins enable engineers to trace faults back to their exact origin by reviewing past events in detail. This comprehensive analysis leads to faster and more accurate problem resolution.
- Data-Driven Decision-Making
With Digital Twins, manufacturers can generate a more accurate representation of their production environment. By analyzing virtual models, teams can assess how changes in machine parameters, workflow design, or maintenance schedules impact overall efficiency. This viewpoint leads to more data-driven decision-making and better long-term process optimizations.
The Future of Data Validation in Manufacturing
As manufacturers strive for greater efficiency, reliability, and scalability, the combination of Fault Learning and Digital Twins presents a transformative opportunity. By integrating these technologies, companies can:
- Automate data validation, reducing reliance on manual processes and minimizing human error.
- Speed up root cause analysis, leading to faster issue resolution and improved uptime.
- Enhance predictive maintenance strategies, preventing costly breakdowns before they occur.
- Ensure compliance with regulatory requirements, improving data accuracy and traceability.
The transition from traditional, labor-intensive validation to AI-driven automation represents a significant leap forward for manufacturing operations. By leveraging these technologies, manufacturers can revolutionize their approach to data validation, ensuring that production environments are optimized for maximum efficiency and future-ready in an increasingly data-driven world.