Beyond Bottleneck Monitoring
The Importance of Comprehensive Data Collection in Manufacturing
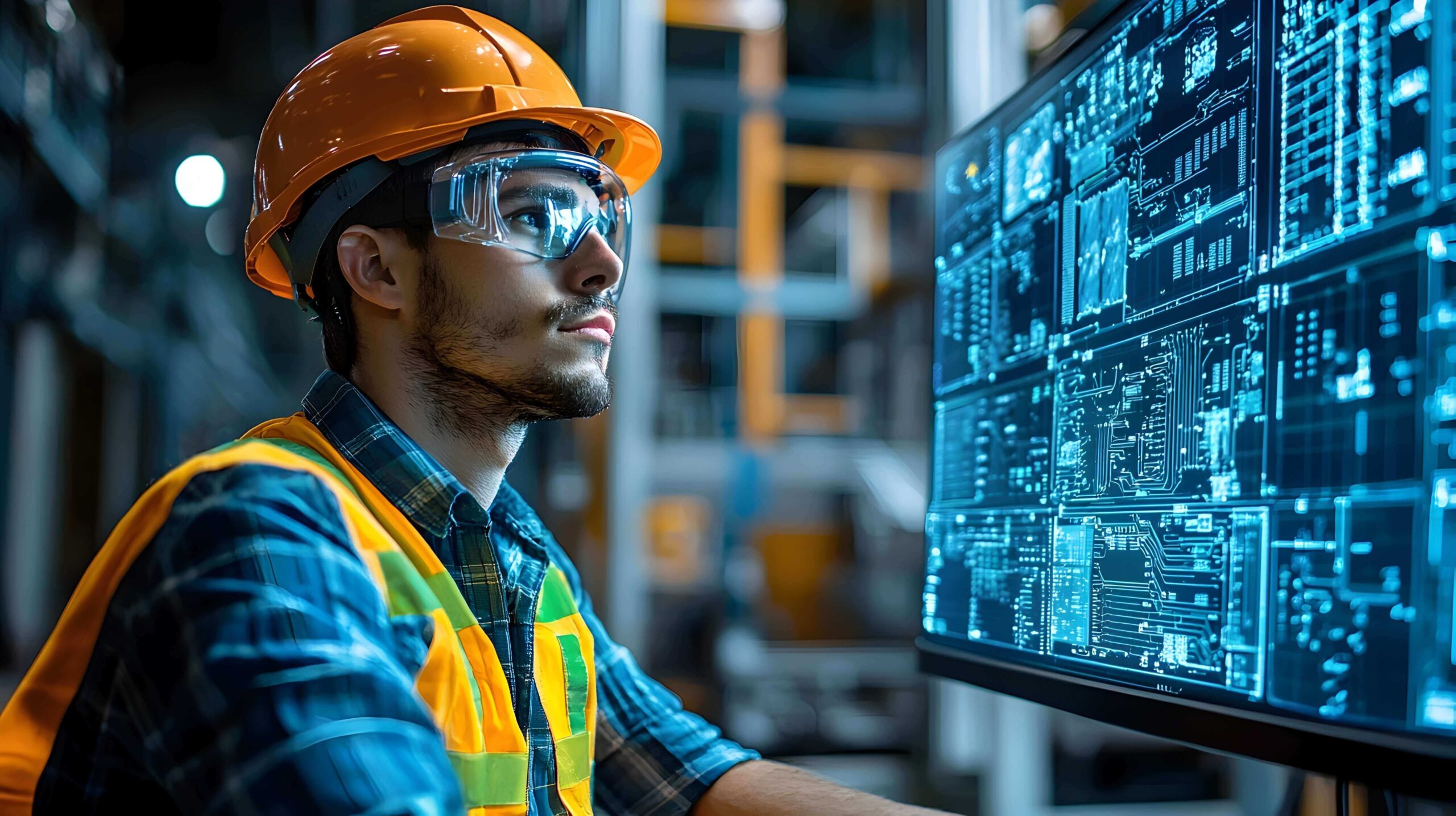
Many manufacturers focus their data collection efforts on bottleneck monitoring and assume that tracking the most constrained point on a production line is enough to optimize efficiency. While identifying and addressing bottlenecks is crucial, this approach alone does not provide a complete picture of manufacturing operations. Limiting data collection to a single point of failure can lead to inefficiencies, undiagnosed performance issues, and missed opportunities for improvement. To fully optimize operations, manufacturers must take a more holistic approach to data collection.
Why Bottleneck Monitoring Falls Short
While bottleneck monitoring provides valuable insights into one point on a production line, it does not capture the full complexity of manufacturing operations. Several factors contribute to inefficiencies that bottleneck tracking alone cannot address:
- Limited Root Cause Analysis
When only the primary bottleneck is monitored, secondary asset failures—such as conveyors, supporting machines, and infeed operations—often go unnoticed. These failures contribute significantly to downtime and production inefficiencies. For example, if a conveyor intermittently stops feeding materials to the bottleneck machine, the machine may appear to be the problem, when the issue actually stems from upstream disruptions. Without a comprehensive data collection system, manufacturers may misdiagnose the root cause of downtime, leading to ineffective corrective actions.
- Parallel Operations Complexity
Many production lines incorporate parallel operations, such as multiple labelers, fillers, or sorters working simultaneously. A failure in one machine may not immediately halt production but can still reduce overall efficiency. Traditional monitoring systems struggle to accurately capture how failures in parallel processes impact production. For instance, in the consumer packaged goods (CPG) industry, a malfunction in one of several labelers may not cause a full shutdown, but it can create imbalances in production flow, leading to inefficiencies that go undetected when only the bottleneck is monitored.
- Lack of Inter-Asset Insights
Manufacturing equipment operates as an interconnected system. A change in one machine’s performance can influence other assets across the production line. Bottleneck monitoring does not provide visibility into these interdependencies, making it difficult to diagnose systemic inefficiencies. Without understanding how different assets interact, manufacturers may implement isolated fixes that fail to address broader operational challenges. A comprehensive data collection approach helps identify patterns and relationships across machines, leading to more effective decision-making and process optimization.
The Benefits of Comprehensive Data Collection
To achieve true operational efficiency, manufacturers must move beyond bottleneck monitoring and implement advanced data collection strategies. A holistic approach ensures that no critical information is overlooked, enabling better decision-making and improved performance. Key benefits of comprehensive data collection include:
- Automated Capture of Minor Downtime Events
Traditional monitoring systems often overlook short, frequent disruptions, yet these seemingly minor events can have a significant cumulative impact on overall equipment effectiveness (OEE). Advanced data collection systems automatically log and analyze these small disruptions, identifying patterns that might indicate larger underlying issues. For example, frequent brief stoppages on a filling machine may suggest minor misalignments or material inconsistencies that, if corrected, could prevent larger breakdowns.
- Enterprise-Wide Data Consistency
For manufacturers operating multiple facilities, consistency in data collection is critical. Discrepancies in how data is recorded across different sites can make it difficult to compare performance, diagnose issues, and implement standardized best practices. Modern data collection systems integrate enterprise-wide analytics, ensuring uniformity in data capture and reporting. This standardization allows manufacturers to benchmark performance across locations, identify trends, and drive company-wide improvements.
- Scalability Through Cloud-Based Architecture
Scalability is a key factor in modern manufacturing data collection. Traditional data collection methods often require significant infrastructure investments, making expansion costly and complex. Cloud-based solutions offer a scalable alternative, enabling manufacturers to increase their data collection capabilities without major hardware upgrades. With cloud architecture, data from multiple machines, lines, or facilities can be aggregated and analyzed in real time, providing a comprehensive overview of operations.
The Path Forward: Implementing a Holistic Data Collection Strategy
To move beyond bottleneck monitoring and achieve a truly great data collection system, manufacturers must embrace emerging technologies that enhance data accuracy, accessibility, and analysis. The following strategies can help build a more advanced data collection framework:
- Utilize Edge Computing for Real-Time Signal Processing
Edge computing enables real-time data processing at the machine level, reducing latency and allowing for faster response times. By analyzing data at the source, manufacturers can detect anomalies and performance issues as they occur, leading to quicker interventions and reduced downtime. Edge computing also minimizes the volume of data that needs to be transmitted to centralized systems, improving efficiency and reducing cloud storage costs.
- Integrate Digital Twins for Validation and Root Cause Analysis
Digital twins—virtual replicas of physical manufacturing systems—allow manufacturers to simulate and test different scenarios without disrupting production. By integrating digital twins with real-time data collection, manufacturers can validate process changes, identify potential failure points, and optimize performance before implementing modifications on the shop floor. This predictive capability enhances root cause analysis, enabling proactive maintenance and process improvements.
- Employ Machine Learning to Optimize Processes Dynamically
Machine learning algorithms can analyze vast amounts of manufacturing data, identifying patterns and optimizing processes dynamically. Instead of relying on static rules for machine operation and maintenance, machine learning-driven systems continuously adapt to changing conditions. For example, predictive maintenance models can anticipate equipment failures based on historical performance data, allowing manufacturers to schedule maintenance before breakdowns occur, minimizing unplanned downtime.
Conclusion
Bottleneck monitoring is a valuable tool, but it is not enough to optimize modern manufacturing operations. To gain a complete understanding of production efficiency, manufacturers must expand their data collection strategies to include all assets, interdependencies, and minor disruptions. By leveraging advanced technologies such as edge computing, digital twins, and machine learning, manufacturers can move beyond traditional monitoring, unlocking new efficiencies, improving OEE, and gaining deeper operational insights. Investing in comprehensive data collection is not just a step toward better efficiency, it is a fundamental shift that enables smarter decision-making and long-term competitiveness across the manufacturing landscape.