The Evolution of Data Collection in Manufacturing
From Manual Methods to Intelligent Systems
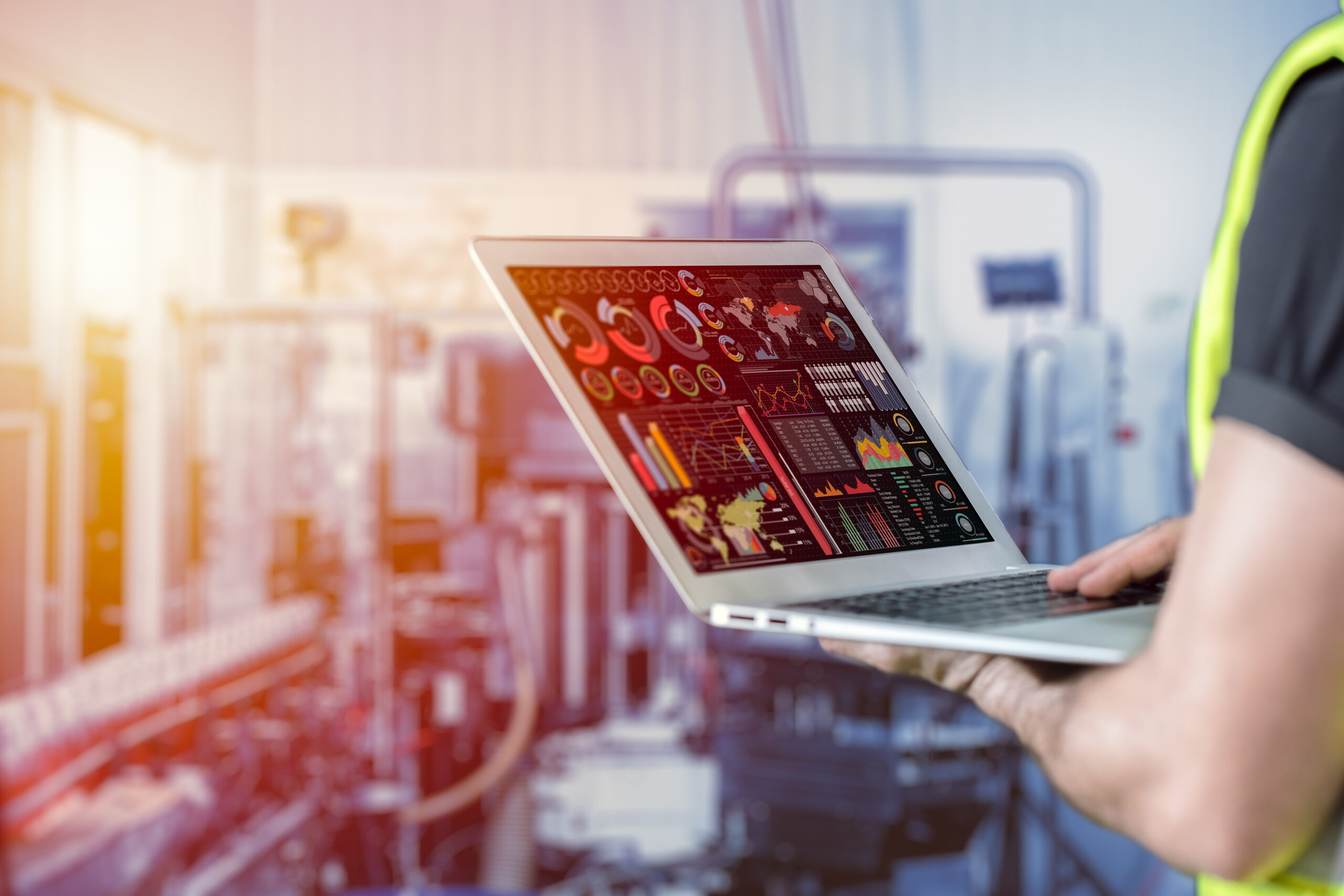
In manufacturing, data collection plays a crucial role in optimizing production processes, minimizing downtime, and improving efficiency. The ability to capture and analyze accurate, real-time data allows manufacturers to make informed decisions, reduce waste, and enhance productivity. However, not all data collection methods are created equal. The evolution from manual data collection to intelligent, automated systems has revolutionized how manufacturers understand and manage their operations, paving the way for higher efficiency and competitiveness in the industry.
The Problems with Manual Data Collection
Manual data collection was once the only way to track production metrics. Operators would record downtime, machine failures, and performance data using spreadsheets, logbooks, or other paper-based methods. While this approach provided some level of insight, it is fraught with significant challenges that limit its effectiveness:
- Inaccuracy
Operators are prone to human error when manually entering data. They may input incorrect information due to fatigue, oversight, or an attempt to simplify reporting. Additionally, they often select downtime reasons based on convenience rather than accuracy, leading to misleading data that does not truly reflect production issues. - Missed Data
Small but frequent downtime events (less than 10 minutes) often go unrecorded. While each instance may seem insignificant, their cumulative impact on production efficiency can be substantial. Over time, these small gaps in reporting can lead to major inefficiencies that go unnoticed and unaddressed. - Limited Visibility
Without a clear, real-time view of the entire production line, operators and managers may misinterpret downtime causes. These misunderstandings could result in ineffective corrective actions that fail to address the root of the problem, leading to recurring issues that hinder overall performance.
The Shortcomings of PLC-Based Data Collection
As automation advanced, many manufacturers turned to Programmable Logic Controllers (PLCs) for data collection. PLCs offered a more structured way to capture machine-level data, reducing the reliance on manual entry. However, this method still has significant limitations:
- Inconsistency Across Production Lines
Different programmers implement PLC logic in various ways, leading to inconsistencies in data collection. This variation makes it difficult to standardize reporting and compare performance across different lines or facilities. Without a unified approach, manufacturers struggle to gain a holistic view of their operations. - Complexity and Cost
Implementing a PLC-based data collection system often requires additional hardware such as data concentrators and network infrastructure. This requirement adds cost and complexity to the manufacturing environment. Additionally, maintaining and upgrading PLC systems requires specialized knowledge, increasing dependency on IT teams and automation engineers. - Validation Challenges in Regulated Industries
In industries like pharmaceuticals, food and beverage, and medical device manufacturing, compliance with strict regulatory standards is mandatory. Revalidating PLC-based systems to meet regulatory requirements can cost anywhere from $500,000 to $1 million, making changes or upgrades a costly and time-consuming endeavor.
The Shift to Intelligent Data Collection
With the rise of Industry 4.0 and the Internet of Things (IoT), manufacturers now have access to advanced data collection solutions that overcome the limitations of both manual and PLC-based methods. These intelligent systems leverage real-time data analytics, machine learning, and automation to provide a comprehensive view of production performance.
Key Features of Advanced Data Collection
- Real-Time Monitoring Across All Assets
Traditional data collection methods often focus on a single bottleneck operation or a few critical machines. In contrast, intelligent data collection systems monitor the entire production line, including conveyors, labelers, cooling tunnels, and auxiliary equipment. This holistic approach allows manufacturers to identify inefficiencies across all assets rather than just isolated points in the process.
- Machine-Level Data Collection for Greater Detail
By gathering data directly from machines and IoT-enabled sensors, manufacturers can capture a dataset that is 5-10 times more detailed than traditional methods. This level of granularity enables deeper insight into machine performance, failure patterns, and process optimization opportunities.
- No-Code/Low-Code Implementation
Unlike PLC-based systems that require extensive programming and integration work, modern intelligent data collection platforms often feature no-code or low-code interfaces. These solutions enable easier deployment, allowing manufacturers to implement and modify data collection processes without extensive IT or engineering involvement. This ease of integration accelerates adoption and ensures a more agile response to changing production needs.
- Fault Learning and Automated Classification
Intelligent systems use machine learning algorithms to automatically detect and categorize faults. Instead of relying on manual input or predefined failure codes, these systems continuously learn from historical data to improve accuracy in fault detection. This automation not only reduces manual configuration but also enables faster response times to production issues, minimizing downtime and increasing overall efficiency.
The Role of loT and Machine Learning in Data Collection
The integration of IoT-driven sensors and machine learning further enhances the capabilities of intelligent data collection systems. IoT-enabled devices collect vast amounts of real-time data, including temperature, vibration, pressure, and machine speed. Machine learning algorithms then analyze this data to detect patterns, predict failures, and recommend proactive maintenance actions.
For example, predictive maintenance powered by machine learning can identify early warning signs of equipment failure before they escalate into costly breakdowns. By addressing potential issues in advance, manufacturers can reduce unplanned downtime, extend equipment lifespan, and optimize maintenance schedules.
The Benefits of Intelligent Data Collection
By transitioning to intelligent data collection, manufacturers can unlock a range of benefits that drive operational efficiency and competitive advantage:
- Increased Accuracy: Eliminates human error and ensures reliable data collection.
- Improved Visibility: Provides a real-time, comprehensive view of production performance.
- Reduced Downtime: Enables proactive maintenance and rapid fault resolution.
- Lower Operational Costs: Minimizes reliance on expensive PLC-based systems and manual reporting.
- Enhanced Decision-Making: Empowers data-driven strategies for continuous improvement.
Conclusion
The manufacturing industry is undergoing rapid digital transformation, and data collection is at the heart of this shift. While manual and PLC-based methods served their purpose in the past, they are no longer sufficient in today’s competitive landscape. Intelligent data collection systems, powered by IoT and machine learning, offer manufacturers the ability to monitor production in real time, capture detailed machine-level insights, and make proactive decisions to improve efficiency.
By embracing advanced data collection technologies, manufacturers can move from reactive to predictive strategies, optimize their operations, and gain a significant edge in an increasingly data-driven industry. The future of manufacturing lies in intelligent data, and those who leverage it effectively will be best positioned for success.